As the automotive industry evolves and embraces advanced technologies like autonomous vehicles and smart transportation systems, the need for high-quality annotated data becomes paramount. Accurate and reliable annotations are crucial for training machine learning algorithms, enabling them to understand and interpret automotive data effectively. In this article, we will delve into the best practices and techniques for data annotation for autonomous vehicles to achieve exceptional results.
Define clear guidelines in the automotive industry
To ensure consistency and reduce ambiguity, it is essential to develop detailed guidelines and instructions for annotators in the auto sector. These guidelines should include examples of both correct and incorrect annotations, as well as explanations of any domain-specific terminology or requirements. By providing clear guidelines, annotators can better understand the expectations and produce accurate annotations.
Ensure understanding of annotation instructions
Proper understanding and adherence to annotation instructions are crucial to avoid wasted efforts and compromised data quality. Start by assigning annotators a small number of easier tasks, allowing them to ask questions and seek clarification. Through iterative training, revise and refine the instructions to be as clear and precise as possible, ensuring consistent and high-quality annotations.
Selecting skilled annotators with automotive expertise
Selecting annotators with expertise in the automotive domain and relevant skills is vital for accurate annotations. Depending on the complexity of the task, additional training may be necessary to ensure annotators fully comprehend the requirements. Expert annotators can bring valuable insights and domain knowledge to the annotation process, resulting in more meaningful and accurate annotations in the automotive industry.
Implement review cycles
Adding a second layer of personnel for reviewing annotations can significantly enhance data quality. These reviewers, chosen from the pool of annotators with proven adeptness at high-quality annotations, oversee the work of the initial annotators. They correct errors, spot and rectify any missed annotations, and ensure consistency across the dataset. Review cycles help identify and rectify mistakes, ultimately improving the overall quality of the annotated data.
Employ a consensus pipeline
A robust quality tactic is to have multiple annotators independently annotate the same data and then determine the correct annotation through consensus. By considering the annotations of multiple individuals, the likelihood of accurately identifying correct annotations increases. In cases where there is consensus among most annotators, the majority annotation can be accepted as accurate. This approach fosters confidence in the data quality and helps eliminate inconsistencies.
Implement quality screening for annotators
To ensure high-quality annotations from the start, it is essential to subject potential annotators to a quality test. Set a predetermined accuracy threshold, such as 99 percent, that annotators must achieve to be eligible for annotation tasks. This quality screening step eliminates the need for rigorous monitoring and ensures a pool of annotators who can deliver reliable and accurate annotations.
Incorporate evaluation tasks
Throughout the annotation process, include evaluation tasks with known correct answers. These tasks serve as benchmarks against which the quality of the annotated data can be assessed. Each annotator should complete the same set of evaluation tasks, allowing for ongoing monitoring of the dataset’s average quality level. Periodically benchmarking the data helps ensure annotators remain on target and provides insights for additional oversight or training if needed.
Leverage automation and AI-assisted annotations
To improve efficiency and accuracy, leverage machine learning algorithms or pre-trained models to assist annotators. Automation can help identify patterns, suggest annotations, and accelerate the annotation process. Human annotators can then validate and refine the results, ensuring the annotations meet the desired quality standards. The synergy between automation and human expertise can significantly enhance the overall efficiency of the annotation process.
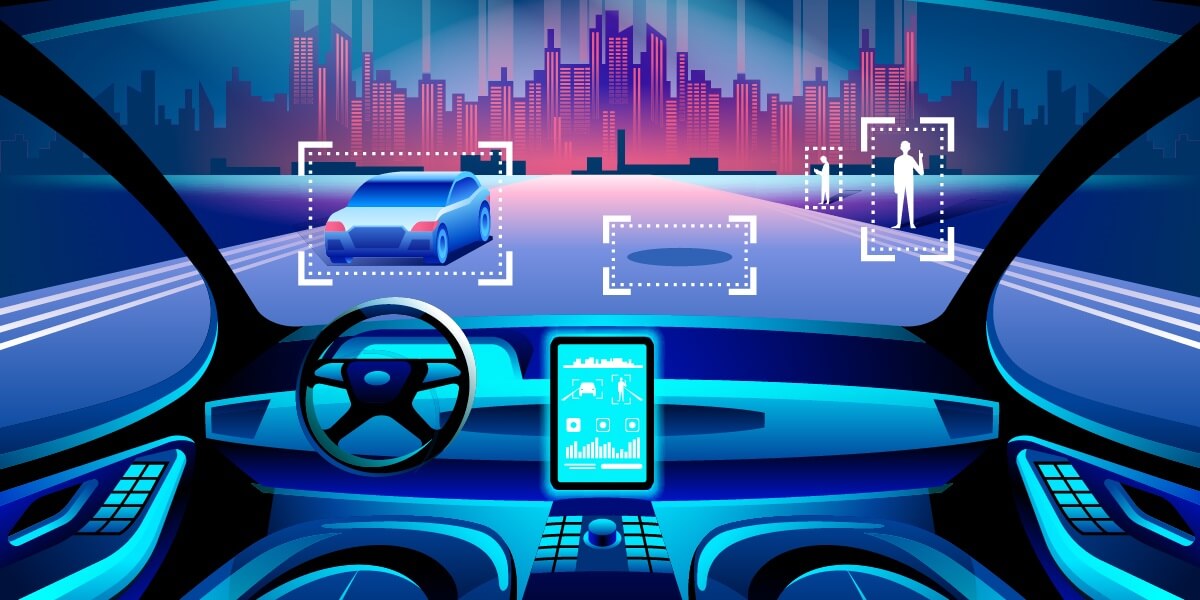
Maintain open communication
Encouraging open communication among annotators, project managers, and ML engineers is crucial for addressing questions, sharing insights, and resolving issues promptly. Open channels of communication foster collaboration, ensuring that everyone involved is aligned with annotation expectations and can contribute their knowledge and expertise to improve the annotation process.
Iterate and refine
The annotation process should be continuously reviewed and updated based on feedback, new insights, or changes in project requirements. Regularly evaluating and refining the annotation process helps ensure its relevance and effectiveness in producing high-quality data. By adapting to evolving needs, the annotation process remains robust and capable of meeting the desired outcomes.
Utilize specialized annotation tools
Employing specialized annotation tools and platforms can streamline the annotation workflow and maintain annotation quality. These tools often provide features such as version control, annotation history, collaboration options, and automated quality checks. Leveraging such tools ensures efficient management of annotations, enables better collaboration among annotators, and helps maintain consistency and quality throughout the annotation process.
Related reading: How data annotation is enhancing machine learning capabilities
Data annotation for autonomous vehicles with precision and accuracy is vital for developing advanced technologies in the automotive industry. With competition rising, it’s not surprising why the global market size for data annotation outsourcing was estimated to be around USD 1.3 Bn in 2020 and is expected to reach USD 4.8 Bn by 2026. Backed by the support of expert data labeling and annotators following the best practices outlined in this comprehensive guide, you can achieve high-quality results in automotive data annotation. Clear guidelines, thorough training, careful selection of annotators, review cycles, consensus pipelines, and leveraging automation contribute to the accuracy and reliability of annotated data. Furthermore, maintaining open communication, iterative refinement, and utilizing specialized annotation tools ensure continuous improvement in the annotation process.
Explore our data annotation tools for autonomous vehicles to build intelligent systems and drive innovation. Contact us to know more.