The rising costs of drug discovery and development and shorter product lifecycles, coupled with greater risks, are affecting the profitability and productivity of life sciences companies. According to research by the Tufts Centre for the Study of Drug Development, the estimated discovery and development cost of each FDA-approved drug is approximately USD 2.6 billion, and it takes about 12-15 years to bring it to market. Additionally, only 10% of drug candidates make it to the market after Phase 1 trials due to procedural roadblocks.
With sales per asset continuing to grow at a slower rate, industry players are using Artificial Intelligence (AI) technologies to streamline drug research and development (R&D) processes, ultimately improving quality and turnaround time across the value chain. According to our research, the startup investment landscape for AI in life sciences R&D will grow to USD 25.4B by 2025, with a CAGR of 41.82% (2021-2025).
AI in life sciences R&D startup funding landscape
While big pharma companies including Johnson & Johnson, AstraZeneca, Bayer, Merck, Novartis, and GSK, have made great strides in adopting AI in pharma applications, the startup landscape in this space is also thriving. AI in life sciences R&D startups have raised a total of USD 10B from 2015 to the first half of 2021.
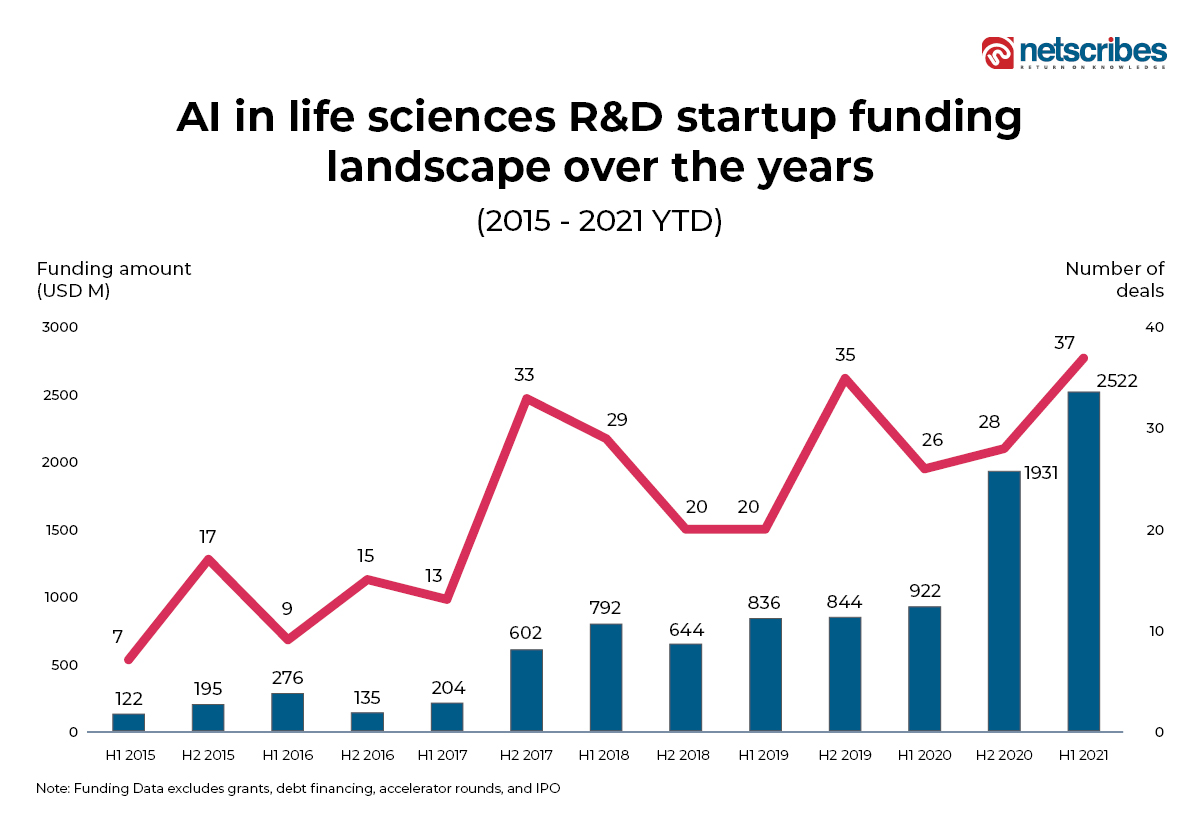
In the first half of 2021, investments flooded into AI in life sciences R&D startups, with their funding increasing by almost 3x compared to the same period in 2020. The startup landscape raked in USD 2.5B (H1 2021). Three major IPOs in this space have already taken place in 2021, including Adgene, Valo Health, and Recursion Pharmaceuticals.
Region-wise funding trends of AI in life sciences R&D
The US leads the world in AI in R&D startup investments, receiving 56% of the global funding in H1 2021. In the Asia-Pacific region, China has received 16% of the funding, while in Europe, the UK has taken in 12.3%.
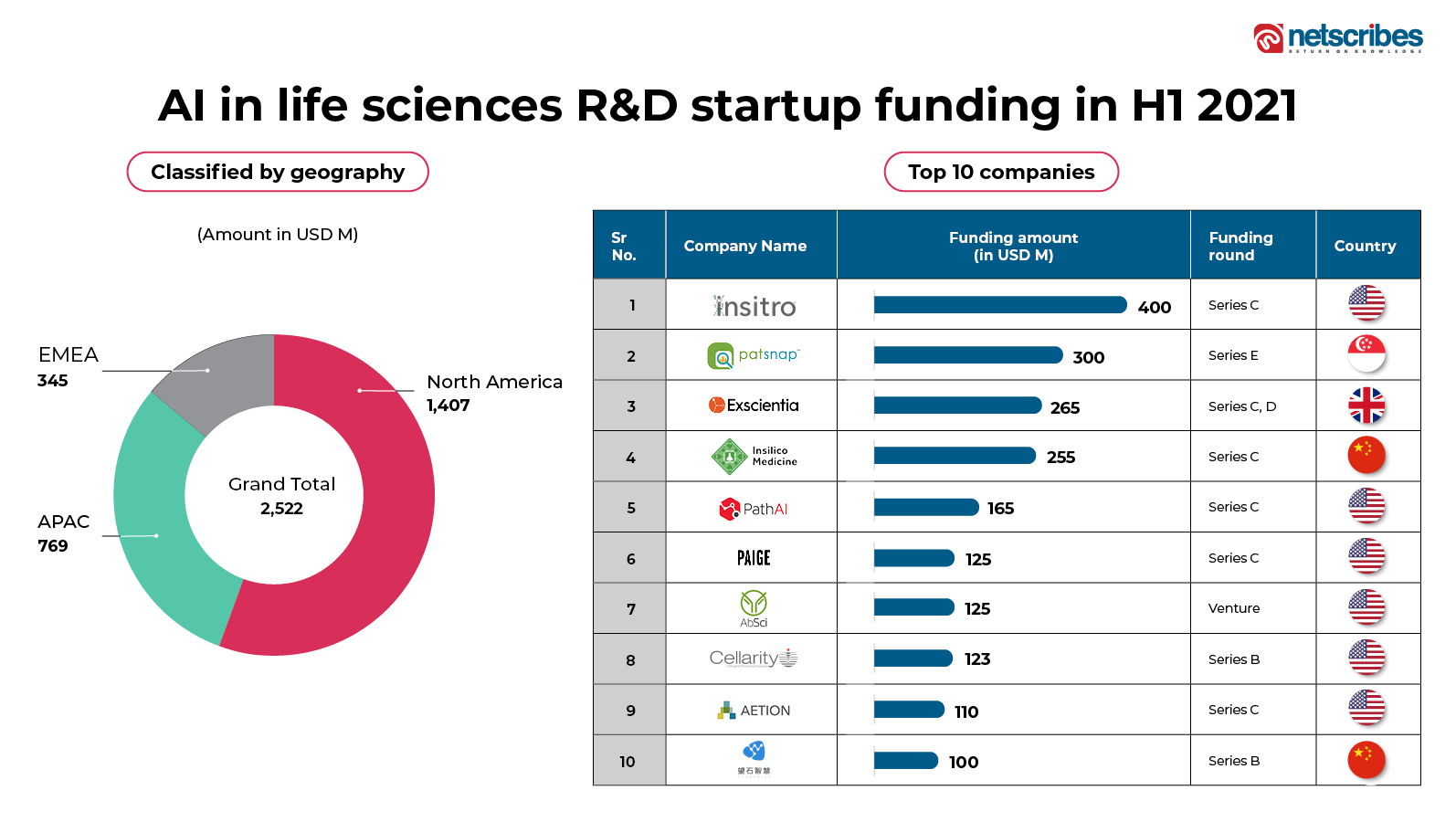
Why is AI in the spotlight?
Growing R&D costs, lengthy R&D cycles, and uncertain success rates are placing downward pressure on the return on investment made by players in the industry. They need to constantly refresh their pipelines and develop novel therapies while simultaneously dealing with escalating R&D operational expenditures.
The drug discovery procedure is typically the most time-consuming, as scientists must conduct thousands of studies before agreeing on the best illness target. Clinical trials, on the other hand, are rife with failure due to human errors in data processing and candidate monitoring.
Using AI in life sciences R&D processes can provide data-driven solutions to long-standing problems. By providing a boost to innovation through improved technology-aided procedures, artificial intelligence is assisting the sector to achieve greater efficiencies. Further, AI-enabled solutions are at the forefront of the industry’s digital transformation due to the need for massive data processing and efficient service delivery.
It is giving companies access to real-world data derived from electronic health records (EHRs), mobile apps, diagnostics, genomics, and data analytics tools, which can aid in the development of personalized and accurate treatment solutions, while significantly lowering the potential for human error. As a result, it can assist the value chain in transitioning from a push paradigm to a combination of push and pull.
Using AI across the stages in the drug R&D cycle
The use of artificial intelligence is being employed at all stages of drug discovery and development, from screening drug compounds to estimating their success rates and assuring accuracy during clinical trials. As well as speeding up the discovery of new therapies, it allows for a more personalized approach to treating patients.
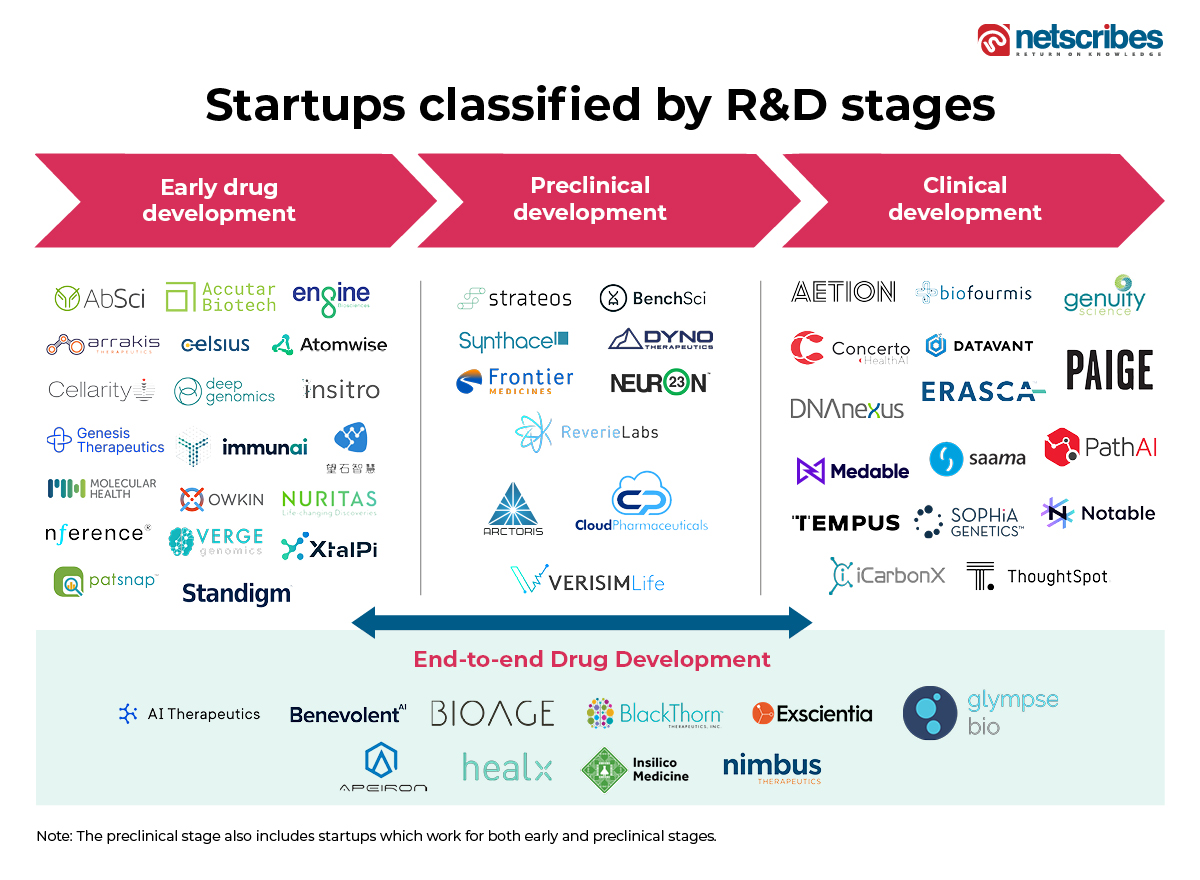
1. Early drug development
The drug discovery procedure involves everything from reading and interpreting existing literature to examining how potential medications react with targets. Applied intelligence can increase drug research success rates by 8-10%, finish it at a quicker rate, and also reduce costs by up to 80% resulting in billions of dollars in savings for the industry.
For instance, PatSnap’s drug research cloud platform used AI to analyze over 114 million chemical structures, clinical trial information, regulatory details, toxicity data, and over 121 million patents and other sources, to expedite the early drug discovery procedure.
2. Preclinical development
Once researchers have found a set of molecules, they must be optimized in terms of the qualities that govern their behavior within an organism. During the preclinical development stage of drug discovery, promising therapeutic targets are tested using animal models. Researchers undertake these trials in-vitro and in-vivo with unrestricted dosages.
Using AI during this phase can help trials run more efficiently and allow researchers to forecast how a drug will behave. It can also help in decoding open and closed-access data on reagents such as antibodies and present published figures with actionable insights, as demonstrated by AI-powered research startup BenchSci.
3. Clinical development
In this stage, researchers begin testing the therapies on human subjects, which is considered the most time-consuming and expensive stage of the R&D cycle. When paired with big data, AI can tackle several important clinical trial challenges by synthesizing and interpreting large data sets.
AI algorithms allow a steady stream of clinical trial data to be cleansed, consolidated, coded, stored, and maintained, while reducing the influence of human error in data collection and allowing for smooth integration with other databases. Berg, a clinical-stage biotechnology startup, for instance, uses AI to analyze data from patient samples in both healthy and diseased states to generate novel biomarkers and therapeutic targets.
Moving forward
While access to data has been made easier due to the COVID-19 pandemic, startups still face many operational challenges, including unsuitable skill sets, cybersecurity concerns, and complex procedures. In the meantime, AI will offer tremendous benefits to drug development and public health as knowledge and experience improve and technology develops.
As the AI in life sciences R&D landscape evolves, companies in this sector must balance the need to safeguard sensitive information with the expanding opportunities afforded by such advanced tools. There must be an open discourse that builds on the lessons acquired from this pandemic and allows everyone — patients, the general public, researchers, and industry – to participate in the future of healthcare.
Fundamental transformation is now required to be successful in the market while establishing a creative and sustainable R&D capability, and organizations must adapt to these evolving market dynamics.
Netscribes’ healthcare market intelligence solutions enable companies in the life sciences industry to get a holistic overview of the current market landscape and stay prepared for emerging trends and technologies. To know more, contact us.