We humans can process 800 words per minute, and it takes us on average 0.6 seconds to name what we see. Forming ideas and inferences based on what someone is saying to us comes easy. As businesses seek greater precision and contextual understanding from their AI and machine learning products, they require them to emulate these simple semantic features of the human brain. But with much of the available data existing in unstructured formats, training AI tools with contextual information is hard. That’s where text annotation services come into the picture.
Research shows that the data annotation tools market size exceeded USD 1 billion in 2021 and is anticipated to grow at a CAGR of over 30% between 2022 and 2028. Images, video, audio, and text – of these formats text is the most popular in terms of application, yet relatively complex to comprehend. Let’s take an example – “They were rocking!” While as humans we would interpret this statement as applause, encouragement, or awe, the typical machine or Natural Language Processing (NLP) model is more likely to perceive the word ‘rocking’ literally, missing its true intent. Here’s where text annotation proves a critical enabler, one on which numerous NLP technologies like chatbots, automatic voice recognition, and sentiment analysis algorithms are founded.
Related reading: How data annotation is enhancing machine learning capabilities
What is text annotation?
Text annotation is nothing but labeling a text document or different elements of its content into a pre-defined classification. Written language can convey a lot of underlying information to a reader, including emotions, sentiments, examples, and opinions. To help machines recognize its true context we need humans to annotate the text that conveys exactly this information.
How does it work?
A human annotator is given a group of text, along with specific labels and guidelines set by the project owner or client. Their task is to map each text with the right labels. Once a sizable number of textual datasets are annotated in this manner they are entered into machine learning algorithms to help the model learn the semantics behind when and why each text was assigned a specific label. When done right, accurate training data helps develop a robust text annotation model enabling AI products to perform better and with little human intervention.
Why do we need it?
Businesses can use text annotation data in a variety of ways, such as:
- Improving the accuracy of responses by digital assistants and chatbots
- Improving the relevance of search results
- Understanding the sentiment behind product reviews and other user-generated content, such as reviews
- Extracting details from documents and forms while maintaining their confidentiality
- Facilitating accurate translation of text from one language to another
Types of text annotation and their applications
1. Text classification
In this type, the entire body or line of text is annotated with a single label. Variants within text classification include:
- Product categorization: To help improve the accuracy and relevance of search results that show up on, for instance, e-commerce sites, this type of annotation proves crucial. Annotators are generally given product titles and descriptions that need to be categorized by choosing from a set list of departments provided by the e-commerce firm. Below is an example.
Source: Schema.org
- Document classification: In this case, the annotator segregates the documents based on a topic or subject. This is generally useful for sectors like education, finance, law, and healthcare that have large online repositories and knowledge platforms requiring a recall of text-based content.
Insurance contracts, bills and receipts, medical reports, and prescriptions are some common use cases of document classification.
Source: ResearchGate
2. Entity annotation
This type of annotation is used in developing robust training datasets for chatbots and other NLP-based platforms. Variants within this type of annotation include:
- Named entity recognition (NER): Annotators of NER are required to label text into relevant entities, such as names, places, brand or organization names, and other similar identifiers. For example, Netscribes helped a leading digital commerce platform improve the efficiency of their AI-powered chatbot by accurately tagging entities in their customer query data. This enabled faster responses, increased conversions, and greater customer satisfaction.
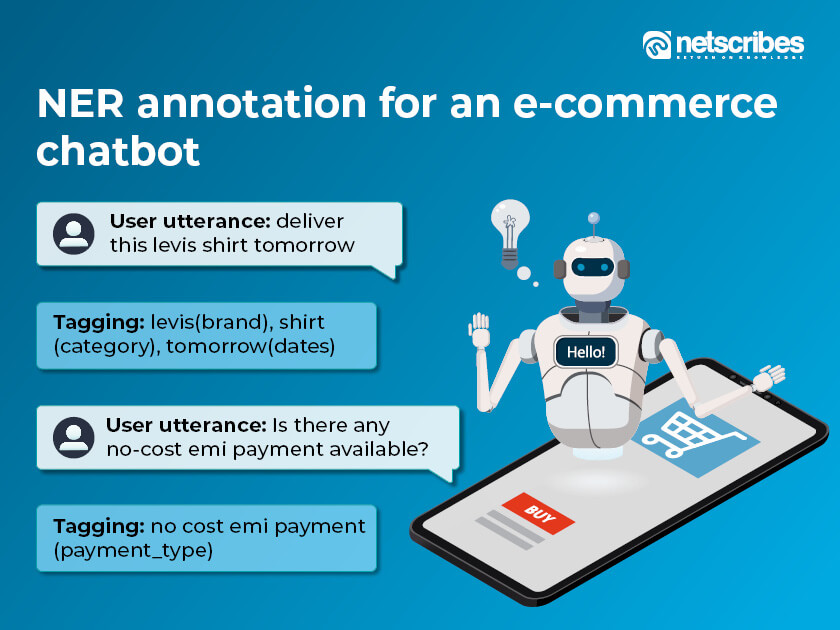
- Part-of-speech (POS) tagging: In this type, annotators are essentially expected to tag the parts of speech in grammar like nouns, verbs, adjectives, pronouns, adverbs, prepositions, conjunctions, etc. This helps digital assistants understand the different types of sentence framing possibilities within a language.
Source: Baeldung
3. Intent annotation
Understanding the underlying intent in human speech is something that machines must be able to identify to be truly useful. For chatbots, if the customer’s intent is not understood correctly, the customer could leave frustrated, or for a business aiming to automate its customer support, it may mean more person-hours invested. That’s why it’s critical for annotators within this type to understand the intent behind a customer’s input, whether in a search bar or chatbot. Here’s an example of the types of classification under intent annotation for a restaurant’s chatbot.
Source: Cloud Academy
4. Sentiment annotation
For any business having a pulse of what customers are saying about its brand, product, or service on online forums is critical. This requires access to the right sentiment data. In sentiment annotation, human annotators are employed to evaluate texts across online websites and social media to tag keywords as positive, neutral, or negative.
Source: AWS
Customer-centric companies often partner with Netscribes to understand not just the broad sentiment from their reviews but a more granular one as depicted above. This helps create strong training data equipped for advanced levels of sentiment analysis. From accurately gauging customer signals to driving personalized responses sentiment annotation finds its use across AI-powered survey tools, digital assistants, and more.
5. Linguistic annotation
This type of annotation is based on phonetics. Here, annotators are tasked with evaluating nuances like natural pauses, stress, intonations, and more, within text and audio datasets to ensure accurate tagging. This approach is of specific importance for training machine translation models, and virtual and voice assistants to name a few.
All in all, to empower AI products to work with precision businesses need accurate and high-quality training data rendered quickly, efficiently, and at scale. It is no wonder savvy brands collaborate with data and text annotation providers like Netscribes to give their customers the best experience while driving higher ROI.
Netscribes provides custom AI solutions with the combined power of humans and technology to help organizations fast-track innovation, accelerate time to market, and increase ROI on their AI investments. To know more about our data annotation solutions, contact us.